What is Enterprise AI, and How Does It Compare to General AI?
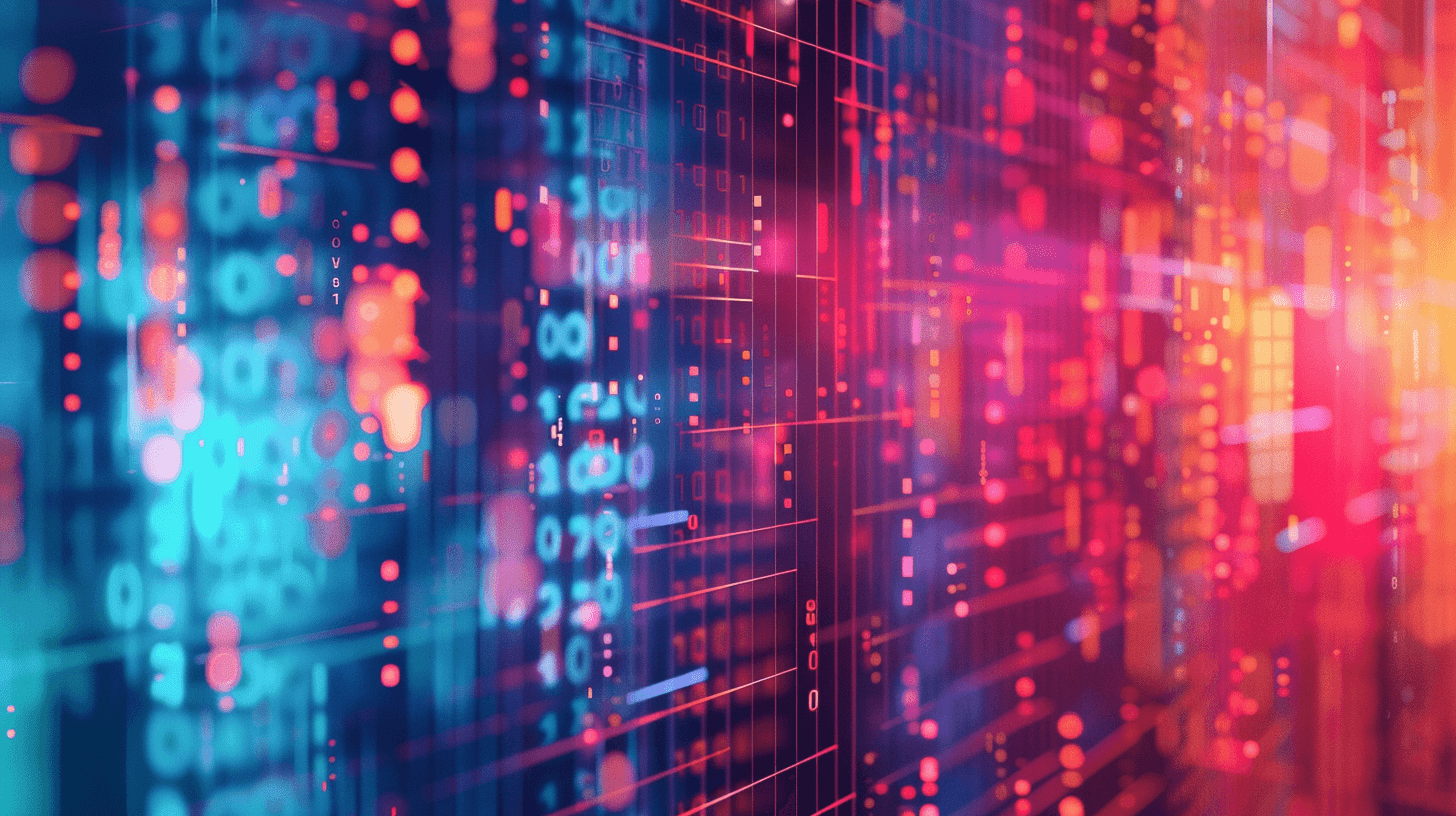
Artificial intelligence (AI) has emerged as a pivotal force driving innovation across industries in today's rapidly evolving technological landscape. Among the various facets of AI, enterprise AI stands out as a powerful tool tailored to meet the unique needs of businesses. But what exactly is enterprise AI, and how does it differ from general AI applications that dominate the consumer market? This comprehensive article delves into the intricacies of enterprise AI, comparing it to general AI and exploring how it revolutionises business operations.
Understanding Enterprise AI
Enterprise AI refers to deploying AI technologies within an organisation's operations to enhance decision-making, automate processes, and improve efficiency. Unlike general AI, which encompasses consumer-focused applications like virtual assistants and recommendation systems, enterprise AI specifically addresses businesses' complex and large-scale needs.
Critical Characteristics of Enterprise AI
-
Scale and Integration: Enterprise AI solutions are integrated into existing business systems and handle large volumes of data across various departments, such as finance, human resources, supply chain, and customer service.
-
Customisation: These AI solutions are highly customised to align with an organisation's unique workflows, data structures, and strategic goals.
-
Data Management: Enterprise AI leverages vast data, necessitating robust data management practices to ensure data quality, security, and compliance.
-
Complexity and Expertise: Implementing enterprise AI involves sophisticated AI techniques and requires collaboration between AI specialists, data scientists, and business domain experts.
-
Regulatory Compliance: Enterprise AI must adhere to industry-specific regulations and standards, including data privacy laws, which can vary by region and industry.
- ROI and Business Impact: Enterprise AI aims to deliver measurable business value, such as increased efficiency, reduced costs, and improved customer satisfaction.
Comparing Enterprise AI and General AI
It is essential to compare enterprise AI's unique benefits with general AI applications prevalent in the market to fully appreciate them. Here, we explore several dimensions where these two types of AI diverge.
Scale and Integration
Enterprise AI
Enterprise AI solutions are developed to integrate seamlessly with existing business systems and processes. They can process and analyse large volumes of data across various departments. For example, an enterprise AI system might integrate with an organisation's ERP (Enterprise Resource Planning) system to provide real-time insights into inventory levels, production schedules, and financial performance.
General AI
General AI applications, on the other hand, are typically designed for individual use or small-scale applications. Examples include virtual assistants like Siri or Alexa, which help users with tasks like setting reminders or searching the web. These applications do not require deep integration with complex business systems.
Customisation
Enterprise AI
Enterprise AI solutions are highly customised to fit a business's needs and workflows. For instance, a retail company might use a customised AI model to predict customer demand based on historical sales data, seasonal trends, and current market conditions. This level of customisation ensures that the AI solution aligns with the business's strategic goals.
General AI
General AI applications are more standardised and offer limited customisation. While they can perform a wide range of tasks, they are not tailored to the unique requirements of individual businesses. For example, a chatbot used for customer service on a retail website may not be as effective as an enterprise AI solution designed specifically for that company's customer service needs.
Data Management
Enterprise AI
Enterprise AI relies on robust data management practices to ensure the quality, security, and compliance of the data it processes. Enterprise applications involve data governance frameworks, data cleansing processes, and compliance with data privacy regulations. For example, a healthcare organisation implementing an enterprise AI solution must ensure that patient data is handled in compliance with HIPAA (Health Insurance Portability and Accountability Act) regulations.
General AI
General AI applications work with less data and do not require the same level of data infrastructure. While they still need to handle data securely, the volume and complexity of the data they process are generally lower than that of enterprise AI solutions.
Complexity and Expertise
Enterprise AI
Deploying enterprise AI involves sophisticated AI techniques, such as machine learning, deep learning, and natural language processing. It requires collaboration between AI specialists, data scientists, and domain experts within the business. For example, a financial institution implementing an enterprise AI solution for fraud detection would involve data scientists who understand AI algorithms and financial experts who understand the intricacies of fraudulent activities.
General AI
General AI applications typically involve more straightforward AI techniques and can be deployed with minimal technical expertise. For example, most consumers can set up a smart home system with AI-powered devices without advanced technical knowledge.
Regulatory Compliance
Enterprise AI
Enterprise AI must comply with industry-specific regulations and standards, which can vary by region and industry; this includes data privacy laws, cybersecurity standards, and industry regulations. For example, a European company implementing enterprise AI must comply with GDPR (General Data Protection Regulation) to ensure the privacy and security of customer data.
General AI
While general AI applications are still subject to regulations, they often face fewer compliance challenges than enterprise AI implementations. For example, a mobile app that uses AI to recommend restaurants faces less regulatory scrutiny than an enterprise AI solution used in healthcare or finance.
ROI and Business Impact
Enterprise AI
The primary goal of enterprise AI is to deliver measurable business value; this includes increasing efficiency, reducing costs, and improving customer satisfaction. For example, a manufacturing company using enterprise AI to optimise its supply chain can achieve significant cost savings and reduce lead times.
General AI
General AI applications may offer convenience and user experience benefits, but the business impact is usually less directly tied to organisational performance metrics. For example, a personal AI assistant can improve productivity for individual users, but its effect on overall business performance is indirect.
Case Study: Retail Industry
Enterprise AI
A large retail company implements an enterprise AI solution to optimise its supply chain. The AI system integrates with the company's ERP system, providing real-time insights into inventory levels, supplier performance, and demand forecasts. The AI system accurately predicts customer demand by analysing historical sales data, market trends, and external factors like weather patterns—enabling the company to optimise inventory levels, reduce stockouts, and minimise excess inventory, resulting in significant cost savings and improved customer satisfaction.
General AI
A small retail business uses a general AI-powered chatbot on its website to assist customers with common inquiries. While the chatbot can handle basic questions like store hours and return policies, it cannot provide personalised product recommendations or assist with complex customer service issues. While the chatbot improves customer convenience, its impact on overall business performance is limited compared to the enterprise AI solution used by the larger retail company.
Case Study: Healthcare Industry
Enterprise AI
A large healthcare organisation implements an enterprise AI solution to improve patient care and operational efficiency. The AI system integrates with the organisation's electronic health record (EHR) system, providing real-time insights into patient data, treatment outcomes, and resource utilisation. The AI system analyses patient data and recommends personalised treatment plans by identifying high-risk patients. This results in better patient outcomes, reduced hospital readmissions, and optimised use of healthcare resources.
General AI
A small medical practice uses a general AI-powered appointment scheduling system. The system can automatically schedule appointments based on availability and send patient reminders. While this improves administrative efficiency and reduces no-shows, it does not provide the same level of clinical decision support or operational optimisation as the enterprise AI solution used by the larger healthcare organisation.
Conclusion
Enterprise AI represents a transformative force in business, offering tailored, large-scale solutions that significantly improve efficiency, decision-making, and customer satisfaction. By integrating seamlessly with existing business systems, managing vast amounts of data, and complying with industry regulations, enterprise AI delivers measurable business value that sets it apart from general AI applications. Through the theoretical case studies in retail and healthcare, we see how enterprise AI can revolutionise business operations, starkly contrasting the more limited scope of general AI. As organisations continue to embrace AI technologies, the distinction between enterprise AI and general AI will become increasingly important, highlighting the unique benefits and capabilities of each.